Posted by AJ Piergiovanni and Anelia Angelova, Analysis Scientists, Google Analysis
Imaginative and prescient-language foundational fashions are constructed on the premise of a single pre-training adopted by subsequent adaptation to a number of downstream duties. Two most important and disjoint coaching eventualities are well-liked: a CLIP-style contrastive studying and next-token prediction. Contrastive studying trains the mannequin to foretell if image-text pairs appropriately match, successfully constructing visible and textual content representations for the corresponding picture and textual content inputs, whereas next-token prediction predicts the most probably subsequent textual content token in a sequence, thus studying to generate textual content, in response to the required job. Contrastive studying permits image-text and text-image retrieval duties, reminiscent of discovering the picture that greatest matches a sure description, and next-token studying permits text-generative duties, reminiscent of Picture Captioning and Visible Query Answering (VQA). Whereas each approaches have demonstrated highly effective outcomes, when a mannequin is pre-trained contrastively, it usually doesn’t fare nicely on text-generative duties and vice-versa. Moreover, adaptation to different duties is usually executed with complicated or inefficient strategies. For instance, in an effort to lengthen a vision-language mannequin to movies, some fashions have to do inference for every video body individually. This limits the dimensions of the movies that may be processed to only some frames and doesn’t absolutely reap the benefits of movement info accessible throughout frames.
Motivated by this, we current “A Easy Structure for Joint Studying for MultiModal Duties”, known as MaMMUT, which is ready to practice collectively for these competing targets and which offers a basis for a lot of vision-language duties both straight or through easy adaptation. MaMMUT is a compact, 2B-parameter multimodal mannequin that trains throughout contrastive, textual content generative, and localization-aware targets. It consists of a single picture encoder and a textual content decoder, which permits for a direct reuse of each elements. Moreover, an easy adaptation to video-text duties requires solely utilizing the picture encoder as soon as and may deal with many extra frames than prior work. In step with latest language fashions (e.g., PaLM, GLaM, GPT3), our structure makes use of a decoder-only textual content mannequin and will be considered a easy extension of language fashions. Whereas modest in dimension, our mannequin outperforms the cutting-edge or achieves aggressive efficiency on image-text and text-image retrieval, video query answering (VideoQA), video captioning, open-vocabulary detection, and VQA.
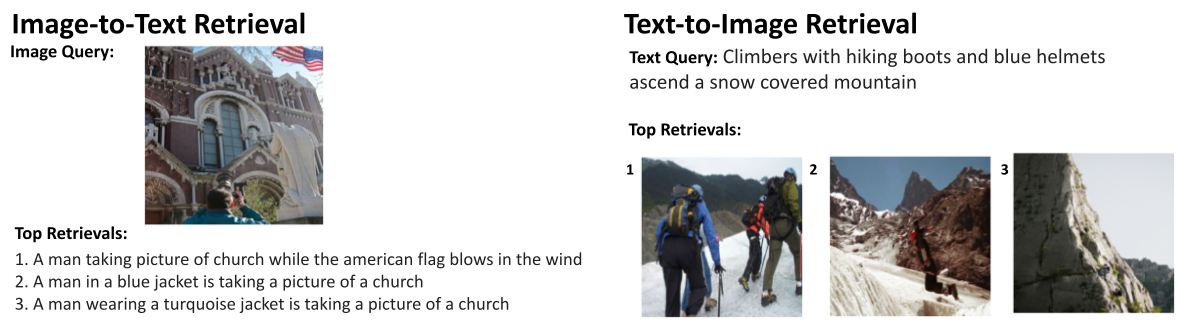 |
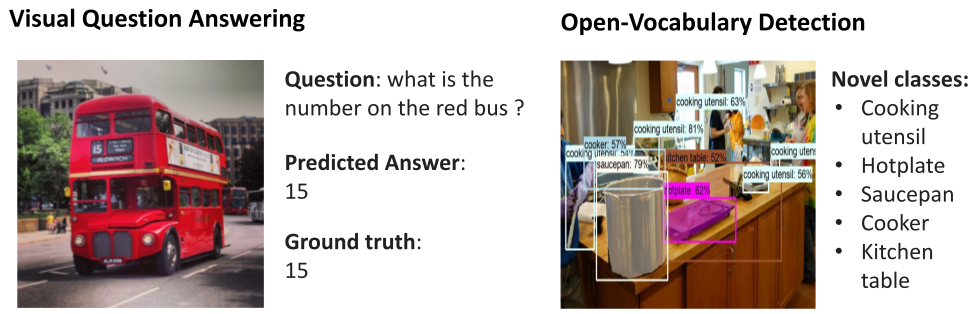 |
 |
The MaMMUT mannequin permits a variety of duties reminiscent of image-text/text-image retrieval (prime left and prime proper), VQA (center left), open-vocabulary detection (center proper), and VideoQA (backside). |
Decoder-only mannequin structure
One shocking discovering is {that a} single language-decoder is enough for all these duties, which obviates the necessity for each complicated constructs and coaching procedures introduced earlier than. For instance, our mannequin (introduced to the left within the determine under) consists of a single visible encoder and single text-decoder, linked through cross consideration, and trains concurrently on each contrastive and text-generative varieties of losses. Comparatively, prior work is both not in a position to deal with image-text retrieval duties, or applies just some losses to just some elements of the mannequin. To allow multimodal duties and absolutely reap the benefits of the decoder-only mannequin, we have to collectively practice each contrastive losses and text-generative captioning-like losses.
 |
MaMMUT structure (left) is a straightforward assemble consisting of a single imaginative and prescient encoder and a single textual content decoder. In comparison with different well-liked vision-language fashions — e.g., PaLI (center) and ALBEF, CoCa (proper) — it trains collectively and effectively for a number of vision-language duties, with each contrastive and text-generative losses, absolutely sharing the weights between the duties. |
Decoder two-pass studying
Decoder-only fashions for language studying present clear benefits in efficiency with smaller mannequin dimension (virtually half the parameters). The primary problem for making use of them to multimodal settings is to unify the contrastive studying (which makes use of unconditional sequence-level illustration) with captioning (which optimizes the probability of a token conditioned on the earlier tokens). We suggest a two-pass strategy to collectively study these two conflicting varieties of textual content representations throughout the decoder. In the course of the first go, we make the most of cross consideration and causal masking to study the caption technology job — the textual content options can attend to the picture options and predict the tokens in sequence. On the second go, we disable the cross-attention and causal masking to study the contrastive job. The textual content options won’t see the picture options however can attend bidirectionally to all textual content tokens without delay to supply the ultimate text-based illustration. Finishing this two-pass strategy throughout the similar decoder permits for accommodating each varieties of duties that have been beforehand arduous to reconcile. Whereas easy, we present that this mannequin structure is ready to present a basis for a number of multimodal duties.
 |
MaMMUT decoder-only two-pass studying permits each contrastive and generative studying paths by the identical mannequin. |
One other benefit of our structure is that, since it’s skilled for these disjoint duties, it may be seamlessly utilized to a number of functions reminiscent of image-text and text-image retrieval, VQA, and captioning.
Furthermore, MaMMUT simply adapts to video-language duties. Earlier approaches used a imaginative and prescient encoder to course of every body individually, which required making use of it a number of instances. That is sluggish and restricts the variety of frames the mannequin can deal with, usually to solely 6–8. With MaMMUT, we use sparse video tubes for light-weight adaptation straight through the spatio-temporal info from the video. Moreover, adapting the mannequin to Open-Vocabulary Detection is finished by merely coaching to detect bounding-boxes through an object-detection head.
 |
Adaptation of the MaMMUT structure to video duties (left) is easy and absolutely reuses the mannequin. That is executed by producing a video “tubes” function illustration, just like picture patches, which can be projected to decrease dimensional tokens and run by way of the imaginative and prescient encoder. Not like prior approaches (proper) that have to run a number of particular person photos by way of the imaginative and prescient encoder, we use it solely as soon as. |
Outcomes
Our mannequin achieves wonderful zero-shot outcomes on image-text and text-image retrieval with none adaptation, outperforming all earlier state-of-the-art fashions. The outcomes on VQA are aggressive with state-of-the-art outcomes, that are achieved by a lot bigger fashions. The PaLI mannequin (17B parameters) and the Flamingo mannequin (80B) have the perfect efficiency on the VQA2.0 dataset, however MaMMUT (2B) has the identical accuracy because the 15B PaLI.
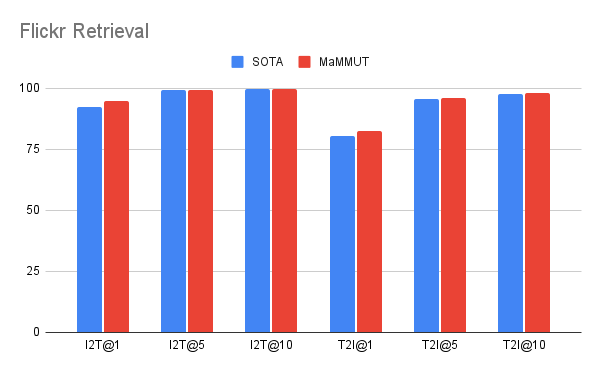 |
MaMMUT outperforms the cutting-edge (SOTA) on Zero-Shot Picture-Textual content (I2T) and Textual content-Picture (T2I) retrieval on each MS-COCO (prime) and Flickr (backside) benchmarks. |
 |
Efficiency on the VQA2.0 dataset is aggressive however doesn’t outperform giant fashions reminiscent of Flamingo-80B and PalI-17B. Efficiency is evaluated within the tougher open-ended textual content technology setting. |
MaMMUT additionally outperforms the state-of-the-art on VideoQA, as proven under on the MSRVTT-QA and MSVD-QA datasets. Notice that we outperform a lot greater fashions reminiscent of Flamingo, which is particularly designed for picture+video pre-training and is pre-trained with each image-text and video-text knowledge.
 |
MaMMUT outperforms the SOTA fashions on VideoQA duties (MSRVTT-QA dataset, prime, MSVD-QA dataset, backside), outperforming a lot bigger fashions, e.g., the 5B GIT2 or Flamingo, which makes use of 80B parameters and is pre-trained for each image-language and vision-language duties. |
Our outcomes outperform the state-of-the-art on open-vocabulary detection fine-tuning as can be proven under.
Key substances
We present that joint coaching of each contrastive and text-generative targets will not be a straightforward job, and in our ablations we discover that these duties are served higher by completely different design decisions. We see that fewer cross-attention connections are higher for retrieval duties, however extra are most popular by VQA duties. But, whereas this exhibits that our mannequin’s design decisions could be suboptimal for particular person duties, our mannequin is simpler than extra complicated, or bigger, fashions.
 |
Ablation research exhibiting that fewer cross-attention connections (1-2) are higher for retrieval duties (prime), whereas extra connections favor text-generative duties reminiscent of VQA (backside). |
Conclusion
We introduced MaMMUT, a easy and compact vision-encoder language-decoder mannequin that collectively trains various conflicting targets to reconcile contrastive-like and text-generative duties. Our mannequin additionally serves as a basis for a lot of extra vision-language duties, reaching state-of-the-art or aggressive efficiency on image-text and text-image retrieval, videoQA, video captioning, open-vocabulary detection and VQA. We hope it may be additional used for extra multimodal functions.
Acknowledgements
The work described is co-authored by: Weicheng Kuo, AJ Piergiovanni, Dahun Kim, Xiyang Luo, Ben Caine, Wei Li, Abhijit Ogale, Luowei Zhou, Andrew Dai, Zhifeng Chen, Claire Cui, and Anelia Angelova. We want to thank Mojtaba Seyedhosseini, Vijay Vasudevan, Priya Goyal, Jiahui Yu, Zirui Wang, Yonghui Wu, Runze Li, Jie Mei, Radu Soricut, Qingqing Huang, Andy Ly, Nan Du, Yuxin Wu, Tom Duerig, Paul Natsev, Zoubin Ghahramani for his or her assist and assist.