A robotic manipulating objects whereas, say, working in a kitchen, will profit from understanding which objects are composed of the identical materials. With this data, the robotic would know to exert a similar quantity of power whether or not it picks up a small pat of butter from a shadowy nook of the counter or a whole stick from contained in the brightly lit fridge.
Identifying objects in a scene which are composed of the identical materials, often known as materials choice, is an particularly difficult downside for machines as a result of a cloth’s look can fluctuate drastically based mostly on the form of the thing or lighting situations.
Scientists at MIT and Adobe Research have taken a step towards fixing this problem. They developed a way that may identify all pixels in a picture representing a given materials, which is proven in a pixel chosen by the person.
The methodology is correct even when objects have various styles and sizes, and the machine-learning mannequin they developed isn’t tricked by shadows or lighting situations that may make the identical materials seem completely different.
Although they educated their mannequin utilizing solely “synthetic” information, that are created by a pc that modifies 3D scenes to produce many ranging images, the system works successfully on actual indoor and out of doors scenes it has by no means seen earlier than. The method may also be used for movies; as soon as the person identifies a pixel in the primary body, the mannequin can identify objects comprised of the identical materials all through the remainder of the video.
Image: Courtesy of the researchers
In addition to functions in scene understanding for robotics, this methodology could possibly be used for picture enhancing or included into computational techniques that deduce the parameters of materials in images. It may be utilized for material-based net suggestion techniques. (Perhaps a client is looking for clothes comprised of a specific kind of cloth, for instance.)
“Knowing what material you are interacting with is often quite important. Although two objects may look similar, they can have different material properties. Our method can facilitate the selection of all the other pixels in an image that are made from the same material,” says Prafull Sharma, {an electrical} engineering and laptop science graduate pupil and lead writer of a paper on this method.
Sharma’s co-authors embody Julien Philip and Michael Gharbi, analysis scientists at Adobe Research; and senior authors William T. Freeman, the Thomas and Gerd Perkins Professor of Electrical Engineering and Computer Science and a member of the Computer Science and Artificial Intelligence Laboratory (CSAIL); Frédo Durand, a professor {of electrical} engineering and laptop science and a member of CSAIL; and Valentin Deschaintre, a analysis scientist at Adobe Research. The analysis will probably be introduced on the SIGGRAPH 2023 convention.
A brand new method
Existing strategies for materials choice wrestle to precisely identify all pixels representing the identical materials. For occasion, some strategies give attention to total objects, however one object may be composed of a number of materials, like a chair with wood arms and a leather-based seat. Other strategies could make the most of a predetermined set of materials, however these usually have broad labels like “wood,” even if there are millions of kinds of wooden.
Instead, Sharma and his collaborators developed a machine-learning method that dynamically evaluates all pixels in a picture to decide the fabric similarities between a pixel the person selects and all different areas of the picture. If a picture comprises a desk and two chairs, and the chair legs and tabletop are manufactured from the identical kind of wooden, their mannequin might precisely identify these similar areas.
Before the researchers might develop an AI methodology to find out how to choose similar materials, that they had to overcome a number of hurdles. First, no present dataset contained materials that have been labeled finely sufficient to prepare their machine-learning mannequin. The researchers rendered their very own artificial dataset of indoor scenes, which included 50,000 images and greater than 16,000 materials randomly utilized to every object.
“We wanted a dataset where each individual type of material is marked independently,” Sharma says.
Synthetic dataset in hand, they educated a machine-learning mannequin for the duty of figuring out similar materials in actual images — but it surely failed. The researchers realized distribution shift was to blame. This happens when a mannequin is educated on artificial information, but it surely fails when examined on real-world information that may be very completely different from the coaching set.
To remedy this downside, they constructed their mannequin on prime of a pretrained laptop imaginative and prescient mannequin, which has seen hundreds of thousands of actual images. They utilized the prior data of that mannequin by leveraging the visible options it had already discovered.
“In machine learning, when you are using a neural network, usually it is learning the representation and the process of solving the task together. We have disentangled this. The pretrained model gives us the representation, then our neural network just focuses on solving the task,” he says.
Solving for similarity
The researchers’ mannequin transforms the generic, pretrained visible options into material-specific options, and it does this in a manner that’s strong to object shapes or various lighting situations.
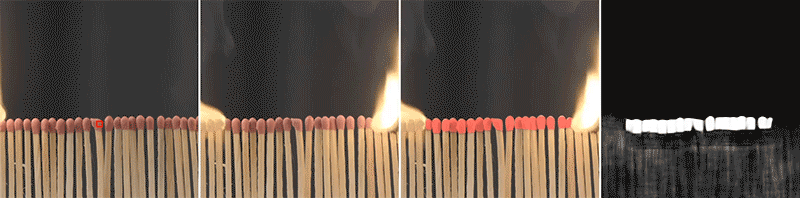
Image: Courtesy of the researchers
The mannequin can then compute a cloth similarity rating for each pixel in the picture. When a person clicks a pixel, the mannequin figures out how shut in look each different pixel is to the question. It produces a map the place every pixel is ranked on a scale from 0 to 1 for similarity.
“The user just clicks one pixel and then the model will automatically select all regions that have the same material,” he says.
Since the mannequin is outputting a similarity rating for every pixel, the person can fine-tune the outcomes by setting a threshold, similar to 90 % similarity, and obtain a map of the picture with these areas highlighted. The methodology additionally works for cross-image choice — the person can choose a pixel in one picture and discover the identical materials in a separate picture.
During experiments, the researchers discovered that their mannequin might predict areas of a picture that contained the identical materials extra precisely than different strategies. When they measured how properly the prediction in contrast to floor fact, which means the precise areas of the picture which are comprised of the identical materials, their mannequin matched up with about 92 % accuracy.
In the longer term, they need to improve the mannequin so it may higher seize fantastic particulars of the objects in a picture, which might enhance the accuracy of their method.
“Rich materials contribute to the functionality and beauty of the world we live in. But computer vision algorithms typically overlook materials, focusing heavily on objects instead. This paper makes an important contribution in recognizing materials in images and video across a broad range of challenging conditions,” says Kavita Bala, Dean of the Cornell Bowers College of Computing and Information Science and Professor of Computer Science, who was not concerned with this work. “This technology can be very useful to end consumers and designers alike. For example, a home owner can envision how expensive choices like reupholstering a couch, or changing the carpeting in a room, might appear, and can be more confident in their design choices based on these visualizations.”