Let’s say you need to prepare a robotic so it understands easy methods to use instruments and might then shortly study to make repairs round your home with a hammer, wrench, and screwdriver. To do this, you would wish an infinite quantity of information demonstrating software use.
Existing robotic datasets differ broadly in modality — some embrace coloration pictures whereas others are composed of tactile imprints, for occasion. Data may be collected in several domains, like simulation or human demos. And every dataset could seize a singular activity and setting.
It is tough to effectively incorporate knowledge from so many sources in a single machine-learning mannequin, so many strategies use only one sort of information to coach a robotic. But robots skilled this manner, with a comparatively small quantity of task-specific knowledge, are sometimes unable to carry out new duties in unfamiliar environments.
In an effort to coach higher multipurpose robots, MIT researchers developed a technique to mix a number of sources of information throughout domains, modalities, and duties utilizing a sort of generative AI often known as diffusion fashions.
They prepare a separate diffusion mannequin to study a technique, or coverage, for finishing one activity utilizing one particular dataset. Then they mix the insurance policies realized by the diffusion fashions right into a basic coverage that allows a robotic to carry out a number of duties in numerous settings.
In simulations and real-world experiments, this coaching strategy enabled a robotic to carry out a number of tool-use duties and adapt to new duties it didn’t see throughout coaching. The technique, often known as Policy Composition (PoCo), led to a 20 % enchancment in activity efficiency when in comparison with baseline methods.
“Addressing heterogeneity in robotic datasets is like a chicken-egg problem. If we want to use a lot of data to train general robot policies, then we first need deployable robots to get all this data. I think that leveraging all the heterogeneous data available, similar to what researchers have done with ChatGPT, is an important step for the robotics field,” says Lirui Wang, {an electrical} engineering and pc science (EECS) graduate scholar and lead creator of a paper on PoCo.
Wang’s coauthors embrace Jialiang Zhao, a mechanical engineering graduate scholar; Yilun Du, an EECS graduate scholar; Edward Adelson, the John and Dorothy Wilson Professor of Vision Science within the Department of Brain and Cognitive Sciences and a member of the Computer Science and Artificial Intelligence Laboratory (CSAIL); and senior creator Russ Tedrake, the Toyota Professor of EECS, Aeronautics and Astronautics, and Mechanical Engineering, and a member of CSAIL. The analysis will probably be introduced on the Robotics: Science and Systems Conference.
Combining disparate datasets
A robotic coverage is a machine-learning mannequin that takes inputs and makes use of them to carry out an motion. One method to consider a coverage is as a technique. In the case of a robotic arm, that technique could be a trajectory, or a sequence of poses that transfer the arm so it picks up a hammer and makes use of it to pound a nail.
Datasets used to study robotic insurance policies are sometimes small and centered on one explicit activity and setting, like packing objects into containers in a warehouse.
“Every single robotic warehouse is generating terabytes of data, but it only belongs to that specific robot installation working on those packages. It is not ideal if you want to use all of these data to train a general machine,” Wang says.
The MIT researchers developed a technique that may take a sequence of smaller datasets, like these gathered from many robotic warehouses, study separate insurance policies from each, and mix the insurance policies in a method that allows a robotic to generalize to many duties.
They symbolize every coverage utilizing a sort of generative AI mannequin often known as a diffusion mannequin. Diffusion fashions, typically used for picture era, study to create new knowledge samples that resemble samples in a coaching dataset by iteratively refining their output.
But slightly than educating a diffusion mannequin to generate pictures, the researchers educate it to generate a trajectory for a robotic. They do that by including noise to the trajectories in a coaching dataset. The diffusion mannequin regularly removes the noise and refines its output right into a trajectory.
This technique, often known as Diffusion Policy, was beforehand launched by researchers at MIT, Columbia University, and the Toyota Research Institute. PoCo builds off this Diffusion Policy work.
The staff trains every diffusion mannequin with a unique sort of dataset, equivalent to one with human video demonstrations and one other gleaned from teleoperation of a robotic arm.
Then the researchers carry out a weighted mixture of the person insurance policies realized by all of the diffusion fashions, iteratively refining the output so the mixed coverage satisfies the targets of every particular person coverage.
Greater than the sum of its elements
“One of the benefits of this approach is that we can combine policies to get the best of both worlds. For instance, a policy trained on real-world data might be able to achieve more dexterity, while a policy trained on simulation might be able to achieve more generalization,” Wang says.
Image: Courtesy of the researchers
Because the insurance policies are skilled individually, one might combine and match diffusion insurance policies to attain higher outcomes for a sure activity. A consumer might additionally add knowledge in a brand new modality or area by coaching an extra Diffusion Policy with that dataset, slightly than beginning your entire course of from scratch.
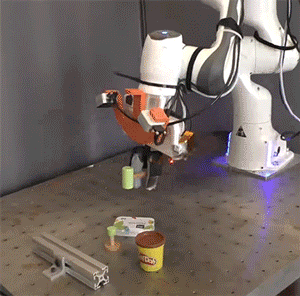
Image: Courtesy of the researchers
The researchers examined PoCo in simulation and on actual robotic arms that carried out a wide range of instruments duties, equivalent to utilizing a hammer to pound a nail and flipping an object with a spatula. PoCo led to a 20 % enchancment in activity efficiency in comparison with baseline strategies.
“The striking thing was that when we finished tuning and visualized it, we can clearly see that the composed trajectory looks much better than either one of them individually,” Wang says.
In the long run, the researchers need to apply this technique to long-horizon duties the place a robotic would decide up one software, use it, then swap to a different software. They additionally need to incorporate bigger robotics datasets to enhance efficiency.
“We will need all three kinds of data to succeed for robotics: internet data, simulation data, and real robot data. How to combine them effectively will be the million-dollar question. PoCo is a solid step on the right track,” says Jim Fan, senior analysis scientist at NVIDIA and chief of the AI Agents Initiative, who was not concerned with this work.
This analysis is funded, partly, by Amazon, the Singapore Defense Science and Technology Agency, the U.S. National Science Foundation, and the Toyota Research Institute.